Looks Smart, Gets It Wrong: Hallucination and AI Agents
Generative AI is impressive — but it makes things up more often than most of us realize.
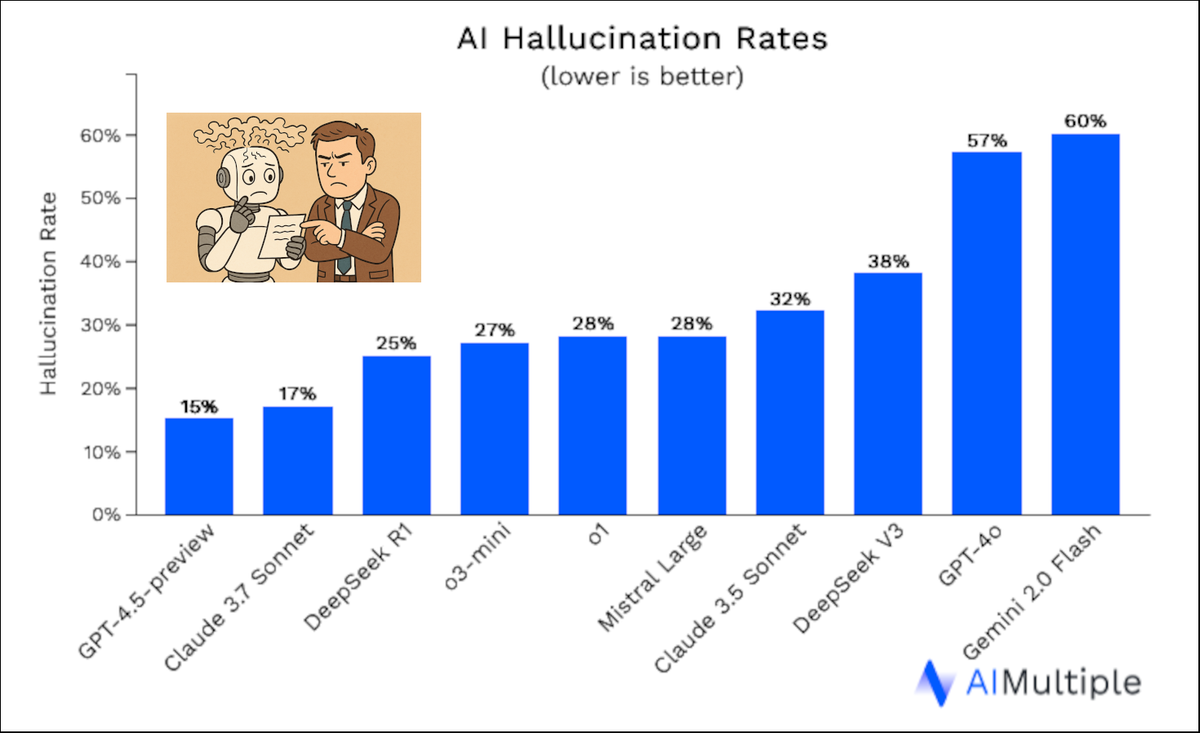
According to recent research by AI Multiple, hallucination remains one of the most persistent risks in commercial AI use. For organizations adopting AI agents to automate tasks and decisions, this isn't a minor issue. For many use cases, it's a deal-breaker
At The Good Agents Company, we believe that one foundation of any trustworthy AI agent is accuracy. If your AI can't be trusted to get the facts right, everything else falls apart.
The Scope of the Problem
AI Multiple highlights that hallucination is more than a glitch — it's common and systemic. Key findings include:
- Hallucination rates reach 15–27% in top LLMs like GPT-4 and Claude 2.
- Even models fine-tuned on specific domains (e.g., biomedical) hallucinate up to 19% of the time.
- Retrieval-Augmented Generation (RAG) reduces hallucinations but does not eliminate them — especially when prompts are ambiguous or data sources are weak.
Put simply: you cannot assume that your AI’s output is grounded in fact. And when that AI is embedded in an agent — making multiple decisions across steps — the risk multiplies.
Agents Amplify Hallucination
A single hallucinated response is bad enough. But agents chain together multiple model calls: asking questions, summarizing results, drafting emails, even triggering actions. Each step relies on the last. A hallucination early on can pollute the entire chain, leading to misleading, harmful, or even unlawful outcomes.
How to Engineer for Accuracy
Accuracy doesn’t come from hoping your model behaves. It comes from constraining tasks, validating outputs, and designing for reliability. The Good Agents Accuracy Checklist outlines what you should expect from any production-grade AI agent:
✅ Limit tasks to those where the “truth” can be verified
✅ Use high-quality retrieval systems, not just model memory
✅ Require traceable sources for every generated claim
✅ Flag uncertainty or low-confidence outputs
✅ Continuously test prompts and outputs against known benchmarks
AI Multiple echoes this guidance: they recommend techniques such as chain-of-thought prompting, answer justification, and post-processing with rules-based filters — all ways to manage hallucination risk in agent design.
What Should Leaders Do?
If you’re exploring or deploying AI agents, here are three questions to ask today:
- What’s the cost of being wrong?
Map out where hallucinations could cause damage — reputational, legal, operational. - How do we test for truth?
Create processes that validate outputs before decisions or communications are made. - Can we prove provenance?
Ensure every answer or recommendation can be traced to its source.
The future of enterprise AI depends not on models that sound smart — but on answers and actions that are the right ones.